The Adoption of Digital Twins in Manufacturing
Last edited on Jun, 05 2024 11:57:24 AM
Reading time: 3 minutes
Written by Hessel Snijder
Table of contents
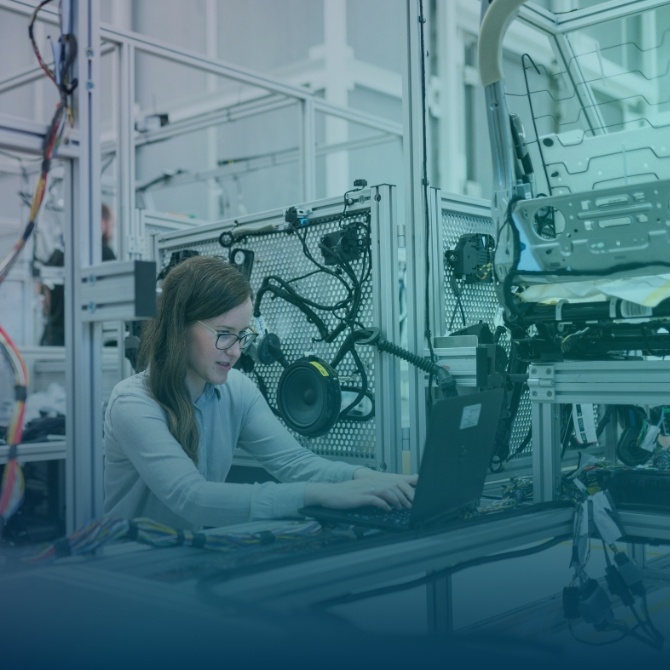
In the relentless pursuit of operational excellence and superior product quality, the manufacturing industry finds itself at the threshold of a technological revolution. At the forefront of this transformation is the adoption of ‘digital twins’, a concept that promises not only to enhance operational efficiency but also to redefine competitive strategies. This article delves into the emergence of digital twins, tracing their historical roots and exploring their potential to bolster the manufacturing sector's competitiveness.
A Historical Perspective: The Genesis of Digital Twins
The origins of digital twins can be traced back to an unlikely historical moment—the Apollo 13 explosion. Faced with the challenge of diagnosing the cause of the explosion from 330,000 km away, NASA pioneered the use of simulation models to digitally analyze the damage. This marked the inception of the digital twin concept, enabling a forensic examination of design flaws through data replication.
What are Digital Twins?
To comprehend the full scope of digital twins, it is crucial to define this technology precisely. A digital twin represents a virtual model of a physical object, person, or process, allowing for simulations to gain insights into its real-world counterpart. Unlike traditional 3D visualizations or standalone simulations, a digital twin provides an accurate, real-time representation, enriched with behavioral insights and visual depictions. It serves as an ideal foundation for product lifecycle management (PLM), extending from creation to disposal, and is designed around the value it brings to specific use cases.
The Role of Digital Twins in Product Design
The digital twin often precedes the physical entity, offering a 'virtual twin' during the creation phase. This enables developers to simulate the entire product lifecycle early in the design process, contrasting with the rigid sequential models of traditional product development. By adopting virtual twins, potential design flaws can be identified and addressed swiftly, thereby saving valuable development time.
Process Analysis Through Digital Twins
A digital twin can also be a replica of a process such as an entire factory, a production line, or machine parts. A digital twin is created by unlocking real-time data from sensors and combining it with computer-aided design (CAD) models and other simulation tools. The primary reason for implementing digital twins is to be able to make data-driven decisions. This can be achieved, for example, by applying predictive maintenance to identify potential problems before they actually occur, or by monitoring and analyzing processes in real-time.
Use cases of digital twins are very diverse. For example, Emixa has set up a digital twin at a plastic packaging manufacturer. The long-term goal: being able to make decisions based on data to increase the quality their products. This started with a proof-of-concept in which data from energy sensors and the ERP system is made available in order to implement real-time energy savings. Employees on the work floor are thus able to act immediately in identified situations for possible energy savings. In later phases, more sensor data will be added to the model to expand the digital twin.
Real-world Applications and Future Outlook
The widespread adoption of digital twins in the manufacturing industry is currently delayed and this can be attributed to several challenges:
- First of all, there is the aspect of a solid data infrastructure. Most manufacturing companies rely on enterprise resource planning (ERP) and product lifecycle management (PLM) systems to manage daily operations. Integrating these systems with digital twin technologies is a complex task, requiring seamless data flows and data connections, which is often associated with high costs and time.
- Another obstacle is that some production processes are difficult to capture in deterministic models. Digital twins are based on the principle of close approximation, but in reality some processes may involve complex, non-linear variables that are difficult to predict.
- In addition, machines often contain enormous amounts of data, but unlocking and effectively using this data can be difficult.
Overcoming these obstacles requires a strategic approach, in which investments in data infrastructure and digital competencies must be translated into concrete financial benefits. For example, various parties are investing in digital twin technology, such as Siemens, General Electric (GE), Microsoft Azure and Amazon Web Services. An interesting example is the collaboration between Siemens, an electronics and electrical engineering company specialized in the manufacturing industry, and NVIDIA, a pioneer in graphics and artificial intelligence. Together they are working on an 'industrial metaverse' to develop photorealistic digital twins on a large scale. Emixa closely follows these developments and responds to possible opportunities this will offer our customers.
Last edited on Jun, 05 2024 11:57:24 AM
Reading time: 3 minutes
Written by Hessel Snijder
Also see..
OverviewHennes de Ridder about modular construction: 'Legoisation'
Hennes de Ridder is an Emeritus Professor of Integral Design at TU Delft. As a 'construction...
Read more ⟶Emixa & Croonwolter&dros Celebrate SAP S/4HANA Implementation Success
EC&O Leader Replaces Aging ERP System, Completing Full-Scale Digital Transformation to Enhance...
Read more ⟶Legal Studio and Emixa Assist the Legal Sector in Digitizing Workflows
The legal sector is known for being traditional and conservative. Digitalization is making strides,...
Read more ⟶